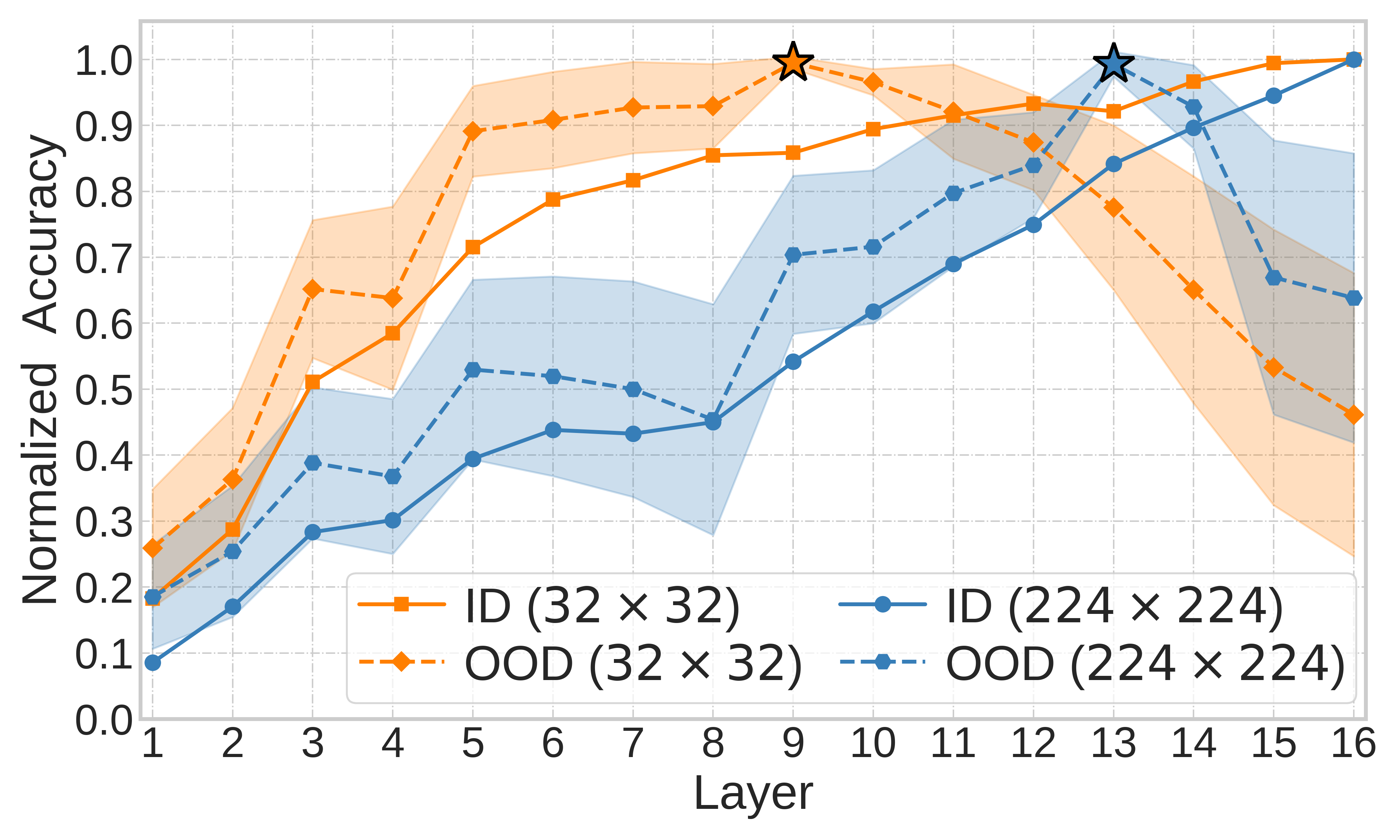
What Variables Affect Out-Of-Distribution Generalization in Pretrained Models?
Md Yousuf Harun, Kyungbok Lee, Jhair Gallardo, Giri Krishnan, Christopher Kanan
Embeddings produced by pre-trained deep neural networks (DNNs) are widely used; however, their efficacy for downstream tasks can vary widely. We study the factors influencing out-of-distribution (OOD) generalization of pre-trained DNN embeddings through the lens of the tunnel effect hypothesis, which suggests deeper DNN layers compress representations and hinder OOD performance. Contrary to earlier work, we find the tunnel effect is not universal. Our results emphasize the danger of generalizing findings from toy datasets to broader contexts.